Temporal Validity is Distinct From External Validity
Because the target is always in the future
I’ve been developing the concept of “temporal validity”: external validity when the known context is in the past and the target context is in the future.
To be clear, this is always the case. Which means that social science is still hamstrung by this guy.
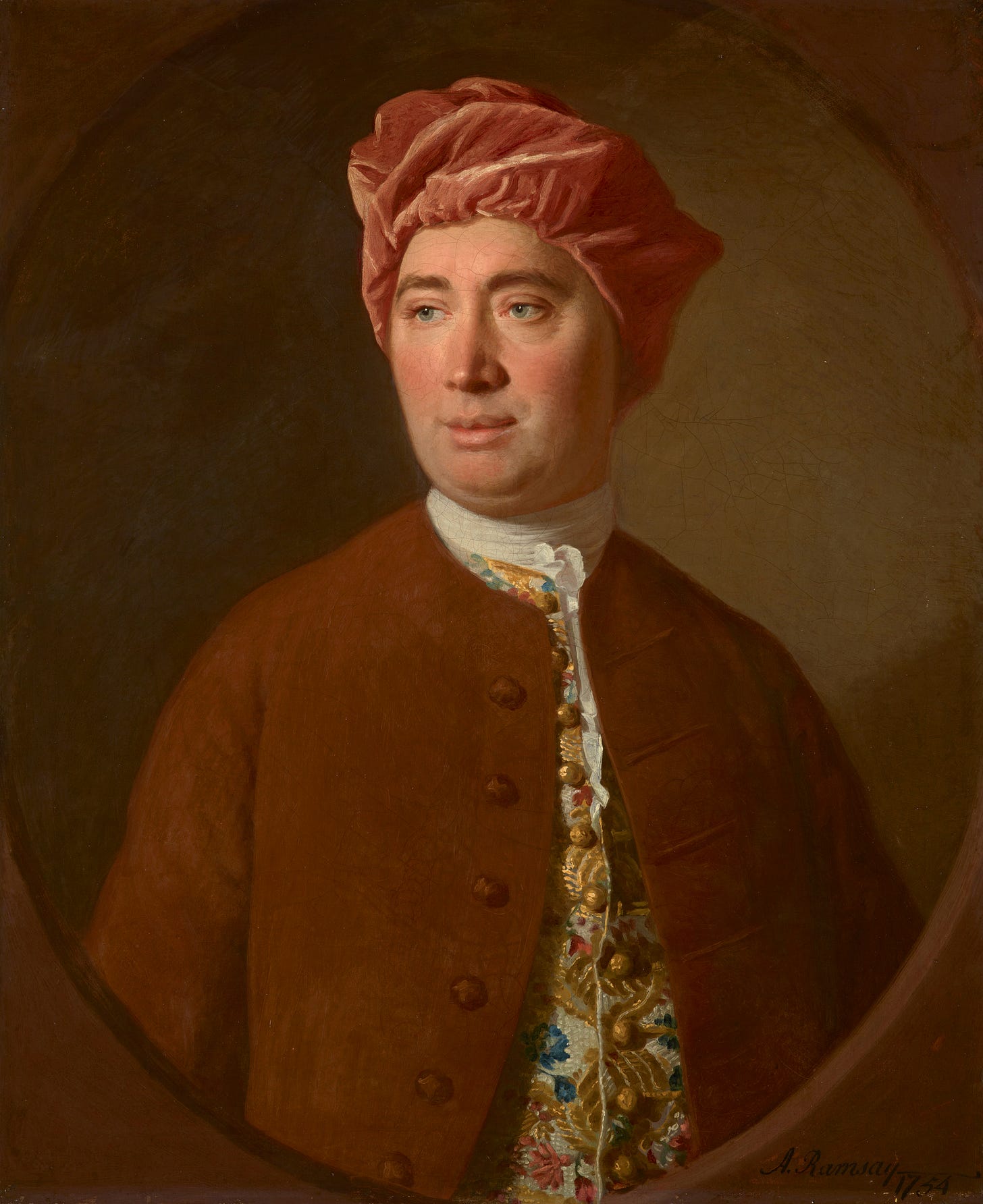
I’ve been working on this problem from several directions, and I have a social science and philosophy of science working paper on the topic. Neither of them nails it; I’ve submitted both and gotten some useful feedback, but this is a really hard problem, and I’m going to keep working on it
Part of the motivation of this blog is to get feedback faster than for journal submissions and a hopefully slightly larger scale than personal emails.
In particular, my first drafts conflate two issues that are better treated separately:
The internet changes everything, very quickly
The target context for the application of knowledge is always in the future
I’ve lots to say about 1, but this post is about 2.
The recent Polmeth saw more attention paid to external validity than ever before. There is a growing sense that the next frontier in social science methodology is external validity; Dan Hopkins said as much in his discussant comments for what I think is the paper that took the most comprehensive look at the problem of external validity: Naoki Egami and Erin Hartman’s Elements of External Validity: Framework, Design, and Analysis.
They identity four components of external validity:
X-validity: pre-treatment characteristics of the units in the sample
Y-validity: outcome measures
T-validity: treatments
C-validity: contexts/setting of experiments
(They correctly note that these dimensions were first described in Campbell and Stanley’s canonical 1963 paper, but the current formalization makes the classic critique newly relevant. They also point out the causal diagram approach to the “contextual exclusion restriction” is developed in Bareinboim and Pearl (2016) . They again do a great service in translating this result from Pearl’s language into the potential outcomes framework most common in social scientific practice.)
This is a far more elegant treatment of something I’ve tried to tackle, and I’ll use it moving forward. I’m most interested in the “Context Validity C”, which I think is the most difficult and which thus requires the strongest assumptions; in fact, for many research questions currently of interest to political scientists, I think these assumptions are always untenable.
Or at least, the amount of causal knowledge we need to make the “contextual exclusion restriction” plausible is orders of magnitude higher than the data we have about “contexts” now. The assumption for C-validity is that contexts only vary (in important ways: they contain different values of treatment mediators) along dimensions that the researcher has knowledge of. That is, the specified “context-mediators” are the only paths along which the context affects the outcome.
The world is too high-dimensional; the curse of dimensionality ensures that real-world C-validity is impossible, at least at present.
(C-validity (and Y- and T-validity) are much more plausible in controlled survey or lab experiments—as long as the target SATE to which you want to generalize is also a controlled survey or lab experiment. If you want to generalize to the real world, you’re back in the realm of needing to adjust for C-, Y-, and T-validity (even if you have a representative sample and thus X-validity), and unless you’re able to do so with the same rigor as you conducted the controlled experiment, that knowledge dissipates in transit.
The case study in which Egami and Hartman apply their framework is a survey experiment where Context is reduced to two dimensions: The United States or Denmark, and whether the ruling coalition in the latter case was center-left or center-right.)
The other issue is that “researchers must define their targets of inference” to establish whether knowledge from a given previous study can be applied to that target. This begs the question.
Indeed, if it is possible to specify the target of inference, researchers are engaging in counterfactual historical research (as in their example of generalizing the results of Broockman and Kalla 2016 to the rest of Florida, or to NYC in 2020). I don’t know why researchers would want to engage in counterfactual historical research. Perhaps this is a useful goal, but it does not seem to conform to mainstream discussions of methodological practice.
If, however, the goal is to statistically inform human decision-making, we have accept the fact that there is a disjunction between all of the temporal contexts in which we have knowledge and all of the temporal contexts in which we want to apply that knowledge.
The target is always in the future! The contemporary “effect-generalizability” [reduced-form, self-contained, agnostic, expert-free, transportability/knowledge synthesis, whatever you want to call it] social scientific paradigm has made significant strides over the past decade, but all of the sophisticated statistical architecture that has made this possible is about to run headfirst into the problem of induction.
Egami and Hartman recognize that writing down the number of assumptions (and the amount of causal data necessary to test them, and the impossibility of knowledge of the future target) makes it clear just how hard the problem of the “effect generalizability” form of external validity is. Hopkins commented, rightly, that the framework gives the impression that RCTs are actually not a useful way to produce knowledge.
As a result, they also develop an alternative framework, outlining the necessary conditions for “sign generalizability”: predicting the sign of the effect of a given intervention in the target context. This is a brilliant move, and the associated proscriptions for research design are invaluable. They propose collecting multiple forms of outcomes (“purposive variations”) to check for robustness in Y-validity, at least in terms of the direction of the effect. This move is already common in large-scale RCTs, but this formalization and the associated sign-generalization test are useful.
Overall, then, we now have tools for dealing with X-validity (sample composition) and Y-validity (outcome validity). T-validity is still a challenge, but it is possible for a portion of research questions. Hovland et al 1949, in seeking to understand the effects of American “educational and ‘indoctrination’ ” films on US Army recruits’ willingness to fight, theorized about the need for the films he studied to be representative of the pool of possible films in order for the treatment effects to generalize.
T-validity is much harder in real-world RCTs. Hotz et al 2005 discusses the idea that what are assumed to be “similar” treatments (here, job training programs in different cities) are in fact dissimilar bundles of subtreatments. The treatment at Site A is (say) a class of size 15, with well-trained instructors, held in the morning, in a classroom equipped with a computer and a projector. The treatment at Site B is (say) a class of size 40, with new instructors, held in the evening, held in a room with a blackboard. The dimensionality of T is high, and without knowledge of how to condition on each of these variables, you don’t have T-validity. Egami and Hartman’s proposed purposive variation works here as well, although it is again much more expensive to get sufficient variation in T than it is to just measure Y in different ways.
And of course we still can’t get C-validity from a single “study” the way that it is usually traditionally conceived. To get purposive variation in C, we need a lot more experiments.
Multi-site experiments like the MetaKeta and Many Labs aim to accomplish purposive variation in context while holding everything else constant. I discuss these valuable initiatives in an earlier post.
The gist is that accepting the need for initiatives like these directly implies that the aggregate amount of social scientific knowledge production has to increase a hundredfold or the range of questions we try to answer has to be sharply curtailed.
If in fact all we can hope to accomplish is sign generalizability, the current allocation of rigor in social science practice is pathological. I’m a fan of Nancy Cartwright’s idea that the chain of causal evidence is only as strong as its weakest link. If the goal of social science is to predict what will happen in response to a given intervention—which I take as the goal of “causal empiricism,” and which is the goal implied by Egami and Hartman—then all of the hard work getting the standard errors right is evaporating in the at-present flimsy final link of the chain.
Instead, I have three proposals. These are “methodological contributions” at the level of aiming to improve the aggregate output of social science knowledge, which I argue is an essential but under-studied level of methodological practice.
We need more: The total amount of social science knowledge produced is radically too small
Look for windfalls: this is why I’ve developed the Upworthy Research Archive, which contains the results of 32,000 RCTs. This more than doubles the number of RCTs in the public domain; we hope that it will help social scientists develop and test models for knowledge synthesis and application to novel contexts.
Establish new institutions to enable massive increases in aggregate social science knowledge production
We need non-causal knowledge: We need more descriptive knowledge at every level in order to make the appropriate adjustments to the target
Much of my recent work has been pure quantitative description
Need to increase the status of pure quantitative description, with associated changes in the publication process
We need to be faster (at least for research on fast-changing subjects like social media): Inductive social science takes time; the world is changing.
This still doesn’t solve the problem of induction!
Smaller time between knowledge generation and the target population (and smaller geographic distance between physical locations) increases the plausibility of C-validity
Lots of progress on open science, online publication